Together with healthcare and legal, the financial sector is one of the slowest adopters of new technologies, mostly due to fraud and identity theft concerns. Some of their operations still rely on legacy, on-premise systems, and the migration towards the cloud environment is slow or not even an option, due to security concerns.
However, using AI in the daily operations of financial institutions can even enhance security by offering constant monitoring of numerous indicators. A more secure environment is a more compliant one. Last but not least enhancing operational excellence is a goal for all modern institutions.
Page Contents
Why should a finance company choose AIOps?
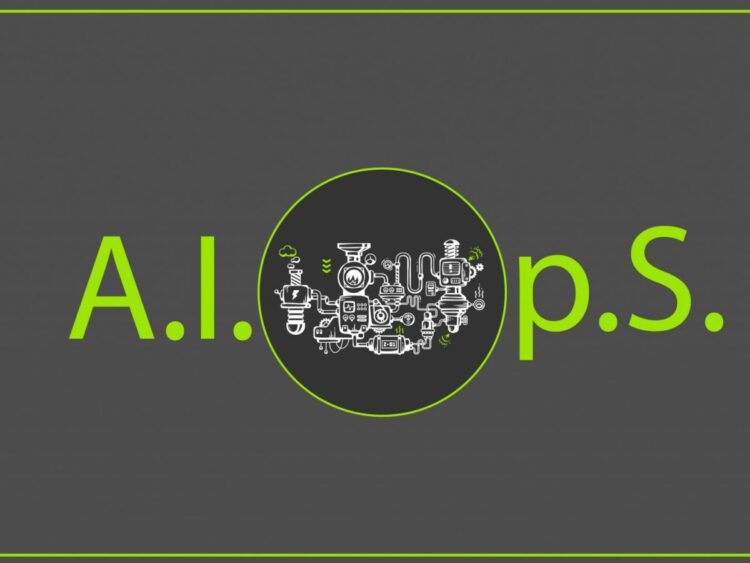
Source: analyticsindiamag.com
The financial sector is overwhelmed with data and information coming from a crowded environment. The challenge is to separate noise from signal and to replace manual practices with automation.
Furthermore, using AIOps helps companies gather data to use in machine learning algorithms in order to get insights about the processes and find ways to solve the most common problems in the company.
Recent advancements in machine learning and AI promise to solve two of this industry’s main issues: fraud detection and compliance problems.
Even more, AIOps can boost these advantages by adopting an agile approach to problem-solving. Other benefits include the timely detection of issues, either predictive or real-time detection, leading to an accelerated mean-time-to-resolution.
AIOps for fraud detection
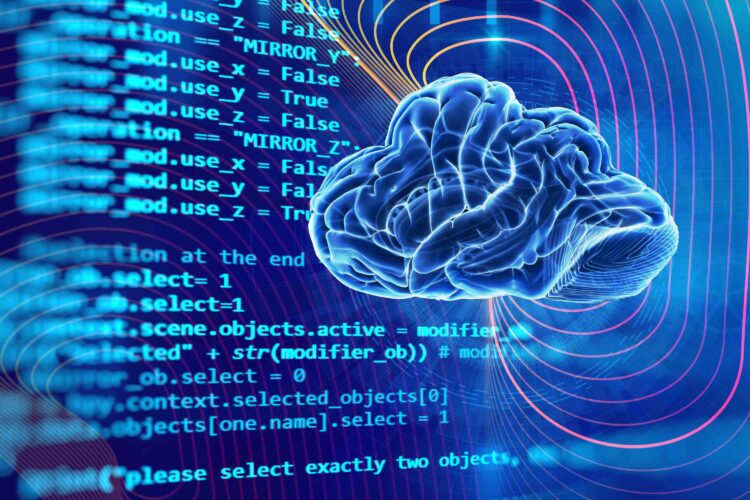
Source: analyticsinsight.net
Although we are living in a very connected world, the time to identify a data breach can be longer than 6 months, thus causing important additional damages. This delay is caused by the sheer volume of data that needs to be processed and the fact that cybercrime is on the rise.
AIOps can speed this process to minutes or seconds through anomaly detection.
Most financial institutions already have in place systems to detect illicit login attempts, large sums transfers, or transfers to countries or accounts which look suspicious.
The advantage of AIOps is that it can put all these together in a comprehensive dashboard and make the connections between the bits of data to decide if the operation is a fraud or a person while traveling for example.
Boosting compliance through AIOps
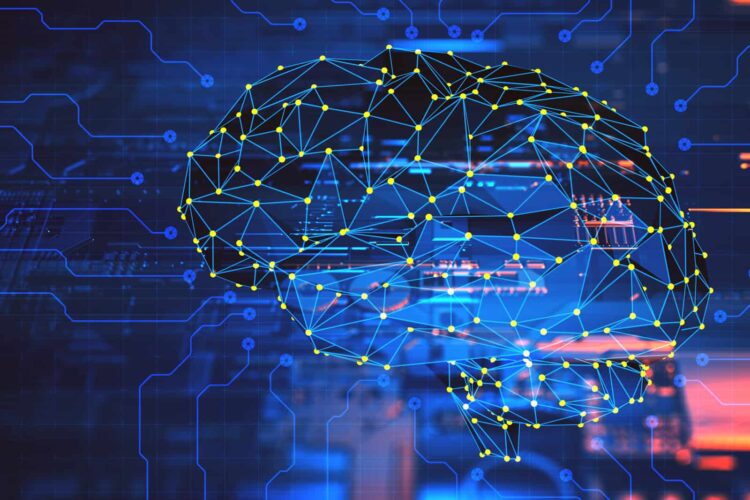
Source: unraveldata.com
IT can strengthen compliance for banks and other financial institutions and it can offer automated ways to manage this aspect. Recent security breaches demonstrate that a weak monitoring infrastructure and not having the right alerts in place can make it very difficult to identify and hedge risks. The outcomes can be extremely negative both from a profit and image perspective.
It is now considered best practice in banks to have a quality assurance department or at least have a dedicated person for this role. However, their tasks are difficult if there is not a way to have a continuous overview of potential problems.
AI can help in every step: from checking that the information which comes into the financial institution’s database is accurate, to handling this data in ways that are in line with security and privacy regulations.
Reduce or Eliminate Downtime
In all high-value companies, every minute of downtime costs thousands of dollars. A recent study by ITIC showed that at the beginning of 2019 the average hour of downtime was $ 100,000, going up to $ 1 million for some companies.
AIOps can help maintain operations running and eliminate this problem by enhancing operations. Downtime can also be regarded as a problem of resource distribution. Therefore AIOps can help with better management to avoid overutilization and create a regulated flow, with no bottlenecks.
Adopting AIOps
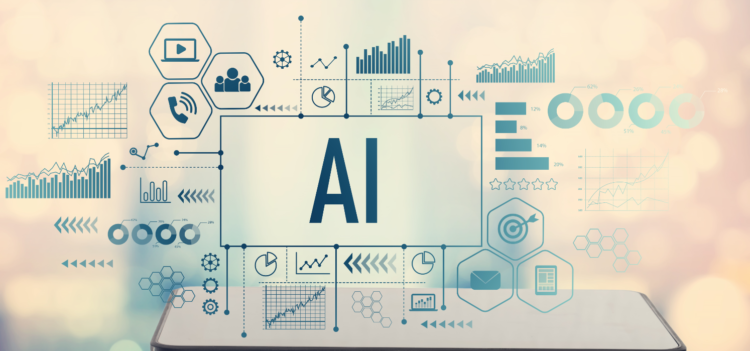
Source: softwarestrategiesblog.com
Although there are numerous benefits for financial institutions to adopt AIOps, the initial setup could be challenging.
First, they need to select those areas and applications which could benefit the most from automation and AI. Gartner suggests starting with just one project before expanding further. This initial project’s success is a good proxy if the organization is ready to implement such a solution on a larger scale and highlights the potential drawbacks.
Secondly, big data, machine learning, and AIOps, as their application, give the organization the ability to use all the data to make the problems visible and actionable. It helps financial institutions withstand sophisticated attacks in real-time and prevent fraudulent information transfer.
Deploying AI-powered solutions can help IT teams reduce their workload, cut costs, and increase efficiency. It is also useful in detecting abnormal activity, which could signal malicious intentions.
Issues with On-premise IT for Financial Services
For the last four decades, keeping servers and applications on-premise was the golden standard in financial services due to security concerns. Right now, this approach has some significant drawbacks: speed, reliability, and scalability. Every time a system crashes, the organization needs to have service teams readily available to firefight issues.
It can take weeks until the operations are back to normal and robust again. There is also the problem of pinpointing the root cause of problems to remove it. This lack of system transparency can result in multiple system crashes, unreliable usage, and frustrated clients.
Reasons to adopt AIOps
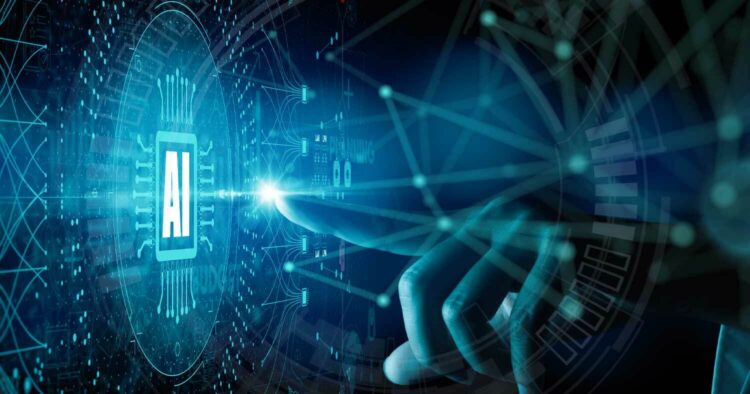
Source: resolve.io
Data streams that could signal a breach are large, change rapidly, and are best analyzed in real-time. Therefore, a solution for fraud detection and prevention should potentially block the attacker before they stand a chance to penetrate the system by a tool which could trigger alerts.
This is the territory where AI and specifically AIOps can make a difference. These tools can help replace an array of operations through the power of pattern analysis powered by machine learning.
A drill-down dashboard holds all the data in place. In the background, the algorithm works with the help of machine learning non-stop to detect anomalies, including bots’ activities, DDOS attacks, failed login attempts, and more.
AI can perform noise reduction, root cause analysis, and performance monitoring, thus reducing data teams’ burden (see siscale.com for more details). They no longer need to look through logs to identify issues and find correlations between them. In an AIOps system, there is the possibility of setting notifications for specific errors and even creating automated responses to fix them without human intervention.